Research 01
教師データ不要の機械学習で、少数サンプルをもとに長大なデータをひも解く
重要なファクターの推定
Unsupervised machine learning is used to reveal information hidden in large data sets using small sample sizes.
Inference of important factors
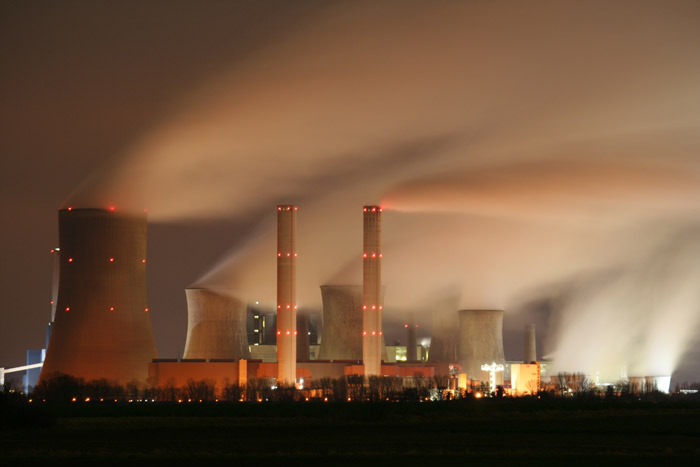
データからコンピューターが自動で学習し、その背景にあるルールやパターンを発見する主流の機械学習では、学習のために膨大な教師データを必要とします。対して、数学をもとにした、テンソル分解という手法を応用する機械学習は、教師データなしの学習によるデータ解析を可能とします。これにより、数万以上のデータを数個のサンプルで解析し、データの中に潜む重要なファクターを、的確に推定することができます。これは、長大な情報を有するDNAと生命現象の関わりの解明にも有効です。(独シュプリンガー社から著書出版)
Mainstream machine learning requires massive datasets with annotations to learn automatically from data and identify hidden rules and patterns. In contrast, using tensor decomposition based on mathematics allows us to analyze data from unannotated data sets. It also enables us to analyze data with tens of thousands of variables with a small number of samples; we can estimate important factors hidden in massive datasets. It is also useful when studying the relationship between life phenomena and DNA, which has a large amount of data (The book was published by Springer, Germany).

この研究が世の中にもたらす可能性
Research Possibilities: what the research create to the World
データマイニング業界
少数サンプルで高次元のデータ解析を必要とする場合に、新たな有効手段を提供し、顧客の拡大に貢献します。
Data mining industry
Even if I only have high-dimensional data from a few samples, I can use it to provide effective methods for increasing customers.
生命科学系研究・開発業界
遺伝子解析など、膨大なデータと時間を要する課題に対し、解決のための新たな手法を提案します。
Life sciences research and development industry
I can offer a novel method for solving tasks that require large datasets and time, such as genomics analysis.
Research 02
情報科学をインシリコ創薬やバイオマーカー探索へ応用する
医学にデジタルで挑む
Using information science to aid in silico drug discovery and biomarker identification.
Challenging medicine digitally
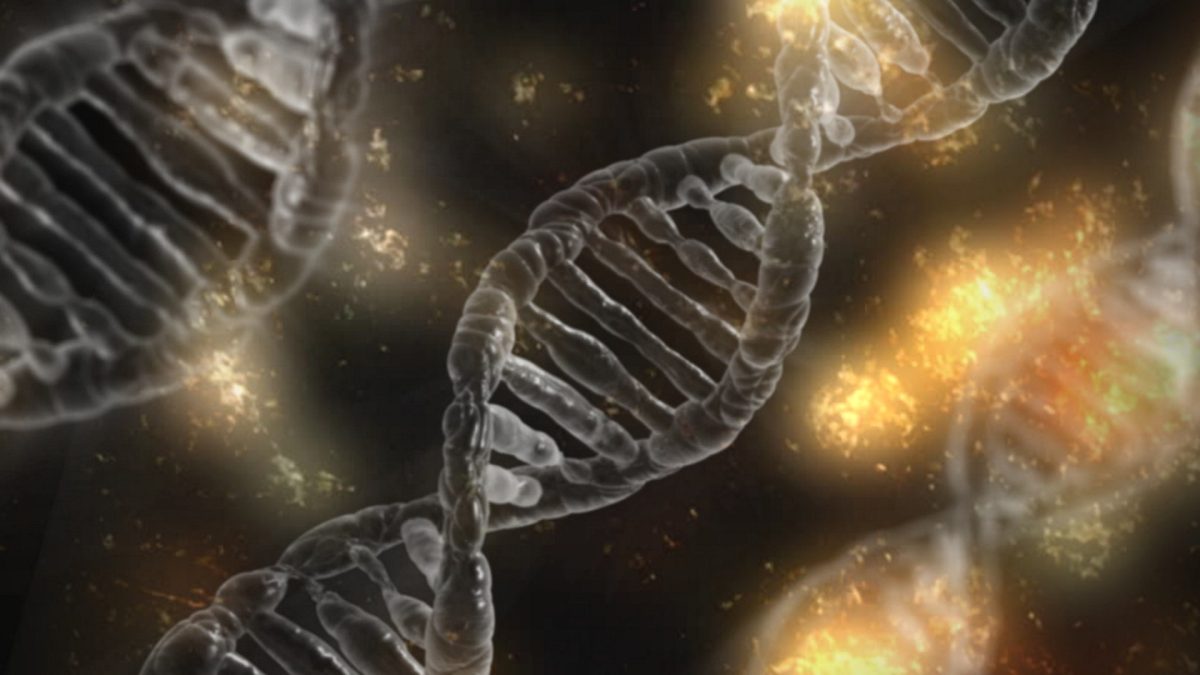
テンソル分解を用いた教師データを必要としない学習手法は、少数のサンプルから高次元のデータ解析が必要な際に有効です。例えば、DNAという長大な情報に潜む特定の疾患の原因遺伝子を、少数の症例から推定するといったゲノム科学は、絶好の標的分野です。臨床医らと協働し、この手法を用いて、遺伝子発現プロファイルを用いたドラッグリポジショニングや、バイオマーカー探索を行っています。
A machine learning method that employs tensor decomposition and does not require annotated datasets effectively analyzes high-dimensional datasets with small samples. The genomic science that infers a small number of disease-causing genes from a long DNA sequence based on a small number of cases is a good example. Using this methodology and collaborating with clinicians, we performed drug repositioning and biomarker identification from gene expression.

この研究が世の中にもたらす可能性
Research Possibilities: what the research create to the World
医療・創薬・化粧品業界
製品やサービス候補になる物質や処置があり、その効果を計測したゲノムデータ(遺伝子発現プロファイルなど)をお持ちの場合、または今後計測の予定がある場合、それらを用いて製品やサービスの改良、新規物質の発見などをサポートすることができます。
Medical/pharmacy /cosmetics industry
We can support the improvement of products and services and the discovery of new materials using genomic datasets (gene expression, for example), which measure the effects of products, materials, and services that may be commercially released.
Research 03
産業への応用も可能、複雑な製造プロセスの問題点を洗い出す
製造プロセスの故障検知
Identifying problems in complex manufacturing processes with industrial applications
Fault detection in manufacturing processes
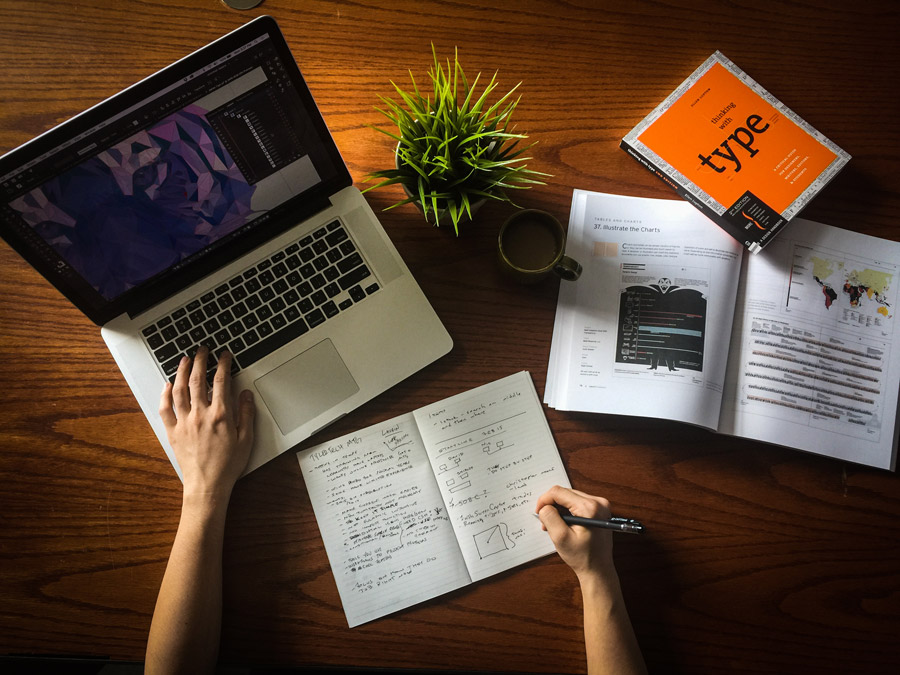
複雑な製造工程を制御することは難しく、不良品が発生して歩留まりが悪くなったとしても、どこが悪いのか原因を発見するのは難しいものです。テンソル分解を用いた教師なし学習を用いれば、複雑な製造工程のどこに問題があるのか、少ない不良品のデータからでも洗い出すことができます。こうした、応用可能な分野の検討も行っています。
It is challenging to control the complicated manufacturing process, and even if defective products occur and the yield decreases, it is difficult to determine the cause of the problem. Using unsupervised learning through tensor decomposition makes it possible to figure out where the problem lies in a complicated manufacturing process, even from a small size of defective product data. I am also examining these potential areas of application.

この研究が世の中にもたらす可能性
Research Possibilities: what the research create to the World
製造業界
製造プロセスが長く、多数のセンサーによる記録はあるが、少数個の不良品が発生するという場合、どのセンサーの値を制御すべきか、どのセンサーの値の異常が不良品に繋がっているかを知ることができます。
Manufacturing industry
If the manufacturing process is lengthy and there are records from many sensors, but there are only a few defective products, then the sensor values that should be controlled and their abnormal values that result in defective products can be determined.
これまでの産学官連携事例
Collaborations
某大手製薬部門
A major pharmaceutical department
2019-
社員教育によるテンソル分解を用いた教師無し学習の技術移転
Technology Transfer of Unsupervised Learning with Tensor Decomposition by Employee Training
某培養細胞開発会社
Certain cultured cell development company
2020/9 –
テンソル分解を用いた教師無し学習を用いた培養細胞改良研究
Cultured cell improvement research using unsupervised learning with tensor decomposition
株式会社アストライアーソフトウエア
Astraea Software Inc.
2021/4 –
技術顧問契約
Technical Advisory Contract
某製造業
A certain manufacturing company
2022/9 –
製造プロセスの解析と改良
Manufacturing Process Analysis and Improvement
株式会社DGCテクノロジー
DGC technology Inc.
2013/1 –
解析技術提供と解析サーバ運用
Analysis technology provision and analysis server operation
実績
Achievements
2021-2022
表彰|Recognition
スタンフォード大学より、バイオインフォマティクスの分野において、上位2%の業績を有すると認定された。
2021.11
講師|Lecturer
行動心理学会・秋の行動計量セミナー「はじめての機械学習」
2021.4
論文|Journal
Y-h. Taguchi & Turki Turki, Mathematical formulation and application of kernel tensor decomposition based unsupervised feature extraction, Knowledge-Based Systems, Vol. 217, 106834 (2021)
経歴
Career
1984
東京工業大学理学部応用物理学科卒業
Tokyo Institute of Technology, Department of Applied Physics, Faculty of Science, Graduate School
1986
東京工業大学大学院理工学研究科物理学専攻修士課程修了
Tokyo Institute of Technology, Graduate School of Science and Technology, Department of Physics, Pre-doctoral Program
1988
東京工業大学大学院理工学研究科物理学専攻博士後期課程修了(理学博士)
Tokyo Institute of Technology, Graduate School of Science and Technology, Department of Physics, Doctoral Program (Doctor of Science)
1988-1997
東京工業大学大学院理工学研究科物理学専攻助手
Assistant Professor, Department of Physics, Graduate School of Science and Technology, Tokyo Institute of Technology
1997-2006
中央大学理工学部物理学科助教授
Associate Professor, Department of Physics, Faculty of Science and Engineering, Chuo University
2006-
中央大学理工学部物理学科教授
Professor, Department of Physics, Faculty of Science and Engineering, Chuo University